|
|
你在逛街买买买,零售商抓紧数据算算算 | Springer Open |
|
论文标题:Discovering temporal regularities in retail customers’ shopping behavior
期刊:EPJ Data Science
作者:Riccardo Guidotti, Lorenzo Gabrielli, Anna Monreale, Dino Pedreschi and Fosca Giannotti
发表时间:2018/03/06
数字识别码:10.1140/epjds/s13688-018-0133-0
原文链接:https://epjdatascience.springeropen.com/articles/10.1140/epjds/s13688-018-0133-0?utm_source=WeChat&utm_medium=Website_linksSocial_media_organic&utm_content=CelZha-MixedBrand-multijournal-Multidisciplinary-China&utm_campaign=ORG_AWA_CZH_BMCWechat_dailyposts_blogs
微信链接:https://mp.weixin.qq.com/s/0LkC5zQAiM0WTK4sQMQiuA
原文作者:Riccardo Guidotti & Anna Monreale
是什么原因引导我们去购买某样东西的呢?近期在EPJ Data Science上发表的一个新研究分析了个人零售消费数据,从而得到了消费者购物随时间变化的档案,由此可以总结出消费者是否会实施购买行为以及何时会购买。这一研究结果显示,消费者可被归类为特定的行为模式和类别,消费者可以据此定制个性化服务,零售商则可以依据个体消费者的购物档案而提供特定商品和折扣。
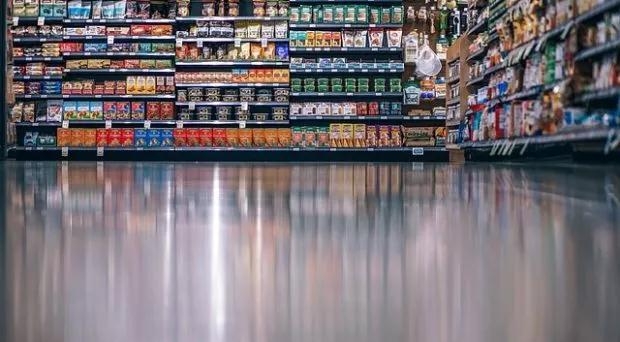
如今我们可以获得海量的零售数据,这些数据使得一些曾经极具挑战性的问题可以通过分析消费者各个方面的消费活动而得到回答。零售数据是一套很复杂的数据,包含多个维度:消费者买哪些东西,买东西的时间和地点,以及与购买行为相关的其他因素,如花了多少钱、买了几样东西等。
要理解消费者如何安排消费时间,那么显然最重要的便是时间维度。本研究利用时间维度作为最主要的构成要素来建立个人购物时间档案。这一档案将消费者的购物时间习惯和其他信息,比如花了多少钱、买了几样东西等联系起来。这些信息有助于按照每个消费者的时间和系统行为定制个性化的新兴市场策略,同时还可以根据消费者的购物时间安排和提升消费者认知来做出一些推荐,并据此推动创新服务。
我们的目标是理解消费者通常在什么情况下进行购买行为,包括是否购买以及何时购买。购物行为中这些不同方面的时间规律哪些更系统化?时间模式的正常顺序是怎样的?
个体及群体时间档案
购物时间档案能够用来描述一个消费者有规律、典型的购物时间行为。每个人的行为都有独特的规律和习惯,这些使他们成为群体中的独特一员。
对于个体的分析能够建立基础,帮助我们理解同样出现在群体层面的普遍规律。据此我们定义了个体和群体时间档案,它能够用于分析消费者消费活动的时间维度。这些模型能够基于消费的时间要素对消费者进行分类,使得我们能够在新的视角下进行个体的探究性分析。
因此,我们得到了每个消费者的以下相关数据:(1)消费者的消费时间档案,包括消费时间记录和顺序,据此可以总结出消费者是否会进行购买以及何时购买;(2)群体角度分析,包括消费者可以与其他人比较和无法比较的部分,由此我们分析出不同消费者的消费模式中共有的规律。

日常消费行为,单次消费行为和偶尔消费行为
这种分析方法用于依据真实数据进行不同的案例分析,基于9.1万名消费者在七年内的零售数据,目的是找到消费者的消费时间模式并将消费者的时间档案分类,将有相似消费时间行为的消费者分到一起。我们提出的这一构架基于不同的时间相关要素将消费者分为多个类别。
特别要指出的是,这项研究依据消费者去购物中心的时间将消费者全体分为三种主要的群体行为:每日消费行为,即每天发生的购买行为;单次消费行为,特点是每周规律进行的主要消费活动;偶然消费行为即占据一小部分的非习惯性消费活动。
分析发现,在单次消费行为中还可以依据消费额进一步分类,包括:花费在50欧元之内的普通消费行为;50到100欧元之间的高消费行为;100欧元以上的超高消费行为。
通过在个体和群体层面分析消费行为的不同数量,我们识别出了两个不同的消费者类别并将其称为:规律消费者和变化消费者。变化消费者表现出多种不同的购物时间行为模式;而规律消费者购物时间行为模式较为单一。最后,我们所发现的多样化购物规律解释了从时间的角度消费者通常是如何综合不同的购物行为模式并遵循的。
摘要:
Abstract
In this paper we investigate the regularities characterizing the temporal purchasing behavior of the customers of a retail market chain. Most of the literature studying purchasing behavior focuses on what customers buy while giving few importance to the temporal dimension. As a consequence, the state of the art does not allow capturing which are the temporal purchasing patterns of each customers. These patterns should describe the customer’s temporal habits highlighting when she typically makes a purchase in correlation with information about the amount of expenditure, number of purchased items and other similar aggregates. This knowledge could be exploited for different scopes: set temporal discounts for making the purchases of customers more regular with respect the time, set personalized discounts in the day and time window preferred by the customer, provide recommendations for shopping time schedule, etc. To this aim, we introduce a framework for extracting from personal retail data atemporal purchasing profile able to summarize whether and when a customer makes her distinctive purchases. The individual profile describes a set of regular and characterizing shopping behavioral patterns, and the sequences in which these patterns take place. We show how to compare different customers by providing a collective perspective to their individual profiles, and how to group the customers with respect to these comparable profiles. By analyzing real datasets containing millions of shopping sessions we found that there is a limited number of patterns summarizing the temporal purchasing behavior of all the customers, and that they are sequentially followed in a finite number of ways. Moreover, we recognized regular customers characterized by a small number of temporal purchasing behaviors, and changing customers characterized by various types of temporal purchasing behaviors. Finally, we discuss on how the profiles can be exploited both by customers to enable personalized services, and by the retail market chain for providing tailored discounts based on temporal purchasing regularity.
阅读论文全文请访问: https://epjdatascience.springeropen.com/articles/10.1140/epjds/s13688-018-0133-0?utm_source=WeChat&utm_medium=Website_linksSocial_media_organic&utm_content=CelZha-MixedBrand-multijournal-Multidisciplinary-China&utm_campaign=ORG_AWA_CZH_BMCWechat_dailyposts_blogs
期刊介绍:
EPJ Data Science (https://epjdatascience.springeropen.com/) covers a broad range of research areas and applications and particularly encourages contributions from techno-socio-economic systems, where it comprises those research lines that now regard the digital “tracks” of human beings as first-order objects for scientific investigation. Topics include, but are not limited to, human behavior, social interaction (including animal societies), economic and financial systems, management and business networks, socio-technical infrastructure, health and environmental systems, the science of science, as well as general risk and crisis scenario forecasting up to and including policy advice.
2017 Journal Metrics
2.982 - 2-year Impact Factor
3.042 - 5-year Impact Factor
1.361 - Source Normalized Impact per Paper (SNIP)
0.943 - SCImago Journal Rank (SJR)
(来源:科学网)
特别声明:本文转载仅仅是出于传播信息的需要,并不意味着代表本网站观点或证实其内容的真实性;如其他媒体、网站或个人从本网站转载使用,须保留本网站注明的“来源”,并自负版权等法律责任;作者如果不希望被转载或者联系转载稿费等事宜,请与我们接洽。